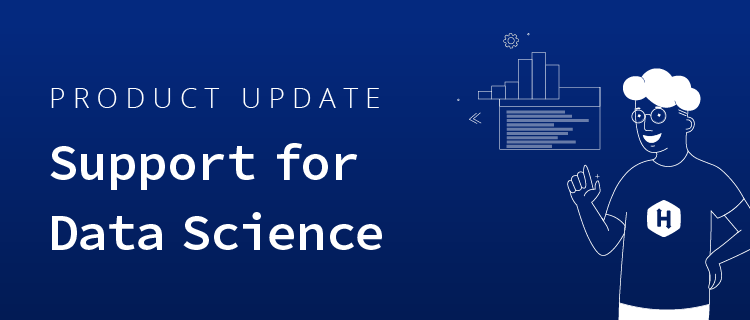
Data science is a rapidly growing field, and one of the most in-demand jobs in the world. As demand for data science candidates grows, identifying and hiring the best candidates is increasingly challenging, and increasingly competitive.
Today, we’re launching HackerRank Projects for Data Science. Previously known as Role-Based Assessments, Projects now gives hiring teams the power to identify and assess top data science candidates through project-based, real world challenges. If you like, you can skip to the details of the launch here. But before we dive into the product details, here’s some background on why we built it:
A brief history of data science talent demand
Since 2013, the market has seen a 256% increase in demand for data science candidates. And it shows no sign of slowing down—in fact, data science roles account for one third of the 15 fastest growing jobs in the United States.
Growth in big data, paired with increased application of data science across industries—from recommendations on TVs to autonomous vehicles—is driving data science demand in every sector.
Big data and analytics are the #1 driver of hiring for technical roles.
And for better or worse, data science talent isn’t in high supply. Take data scientists, for example, who make up only about 2% of the tech talent population (based on self-reported categorizations). To keep up with demand, hiring teams have to look to non-traditional backgrounds—which will require them to have a strong understanding of data science skills.
Understanding distinct data science roles and skills
Despite high and growing demand, data science skills aren’t well understood, due in part to the immaturity of the field.
Since data science is still a young field, the career path of a typical data scientist is anything but linear. Instead, the majority of data science candidates come from a variety of educational backgrounds, from physics, to biology, mathematics, social sciences, and more. And more than 60% of data scientists report learning data science skills outside of a university.
That diverse set of backgrounds makes it hard for hiring teams to define what skills, exactly, a data science candidate needs to be successful. Paired with the competitive talent pool, it’s not surprising that data science roles make up 3 of the top 10 most challenging roles to hire, according to tech recruiters:
Machine Learning Engineers, Data Scientists, and Data Engineers make up 3 of the top 10 most difficult roles to hire.
To complicate things further, the terms “data scientist,” “artificial intelligence engineer,” and “machine learning engineer” are often used interchangeably. This adds another layer of confusion for those trying to understand and recruit for various data science role, all of which demand distinct skill sets.That confusion means companies struggle to identify individuals with strong data science skills—and miss out on quality candidates as a result.
To ease these challenges, we decided to dive deep on what skills, exactly, make a great data scientist. More specifically, we designed a means to evaluate the key skills required in strong data scientist candidates:
Introducing HackerRank Projects for Data Science
To help our customers better identify strong data scientists, we’re introducing HackerRank Projects for Data Science. Here’s what it includes:
New real-world challenges to assess key data science skills
We created a new set of real-world challenges that focus on assessing the key skills strong data scientists need. These include:
- Data Wrangling
- Modeling
- Data Visualization
- Machine Learning
In addition to these new challenges, we’ve created challenge-specific scoring rubrics to simplify candidate review for each skillset. Here’s how we evaluate candidates against each of these new skills:
Data wrangling
Otherwise known as data munging, this set of challenges focuses on evaluating the candidate’s ability to programmatically transform raw data into a more usable format. We evaluate data wrangling skills by testing the candidate’s knowledge on data cleansing, transformation, imputating, summarization, normalization, and outlier/anomaly detection.
Modeling
Building models, or, modeling, primarily refers to the candidate’s ability to build models that effectively answer business questions. This skill tests their ability to leverage processes like feature selection, pattern recognition, and predictive modeling to build models and their ability to evaluate models.
Data Visualization
Above all, a great Data Scientist needs to be a great storyteller—so data visualization is key. This skills evaluates the candidate’s ability to create data visualizations for the purposes of data exploration and storytelling. It tests the candidate’s ability to create data-driven visualizations that clearly convey the story they need to share.
Machine Learning
This skill refers to the candidate’s ability to leverage machine learning to identify and understand meaningful patterns in a dataset. More specifically, HackerRank Projects evaluates candidates’ proficiency in selecting, using, and optimizing machine learning models such as decision trees, random forest, k-nearest neighbors, Naive Bayes, and k-means clustering.
Better candidate experience through Jupyter support
In addition to support for key data science skills, we’ve also made improvements to our HackerRank Projects platform to create a better experience for data science candidates.
To do this, we built support for Jupyter, the most widely used environment in the data science community.
Now, candidates can use an embedded Jupyter development environment for solving data science challenges within HackerRank. This data science-specific environment makes it easier for candidates to showcase their skills in a familiar setting. Since Jupyter is built specifically for data scientists, this option makes for a great candidate experience.
About HackerRank Projects
Data science support is made possible by HackerRank Projects, which uses project-based, real-world challenges to empower hiring teams to assess advanced skills and identify top candidates. Learn more about Projects here, or try a sample test here.
If you want to learn more about HackerRank Projects for Data Science, we invite you to join us for our upcoming data science webinar. We’ll cover best practices for assessing data scientists, including how to leverage HackerRank projects to assess for data wrangling, building models, data visualization, machine learning, and more:
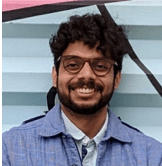
Shikhar Jaiswal is a developer turned Product Manager at HackerRank. He and his team leverage their rich background in software engineering to create great developer experiences within the HackerRank platform.